My general areas of research are data mining, people analytics with the application in intelligent talent management. In general, intelligent talent management refers to a holistic approach for optimizing human capital which enables an organization to fulfill its short- and long-term goals. With the deepening of digitalization within organizations, many work-related activities are recorded and tracked by systems such as the data from Enterprise Resource Planning (ERP) and other online professional platforms, which I call talent data. These resources give us huge opportunities to analyze the employees and organizations in new perspectives. The data mining techniques enable us to refine workflows and paradigms related to talent management, in a more objective, efficient, automatic, and economical way. Specifically, I focus on developing efficient and effective techniques for analyzing talent to meet various intelligent talent management needs. I have addressed two main issues related to talent management. One is job mobility prediction for understanding job hopping patterns. The other is job salary benchmarking for automatically determining the salary for organization’s actual and perspective employees. I have published various research papers including a paper at the prestige conference ACM SIGKDD International Conference on Knowledge Discovery and Data Mining (KDD’ 19) in the research track as the first author.
Talent Job Mobility Prediction:
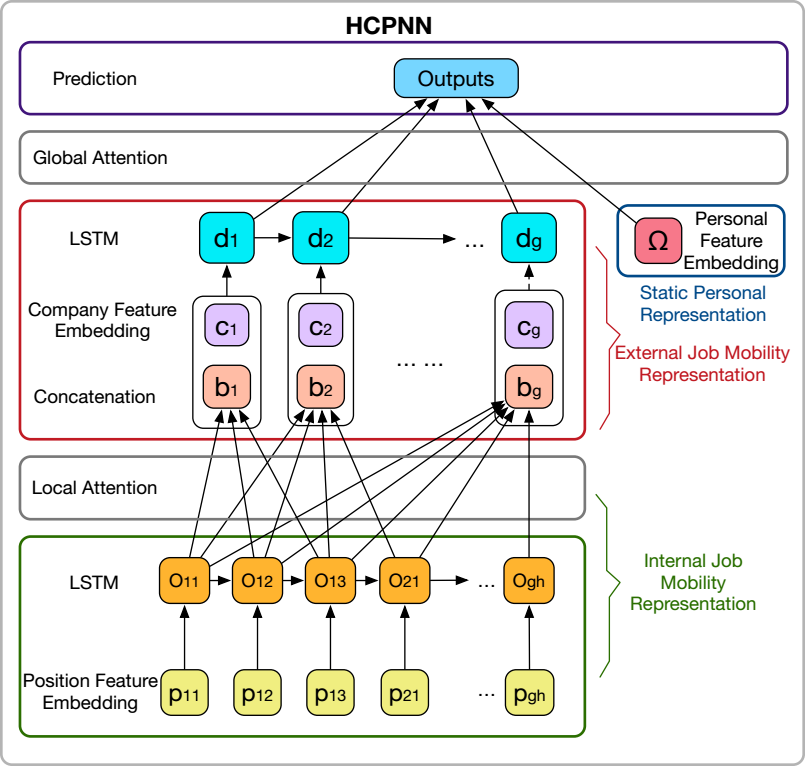
The understanding of job mobility can benefit talent management operations in a number of ways, such as talent recruitment, development, and retention. Understanding the potential career paths of an employee would help executives and department managers in internal promotion decisions to motivate key talents and reduce turnover rate. Also, during the recruiting process, employers may be interested in knowing the probability for candidates to accept job offers. Meanwhile, if there is a high chance of successful hiring, how long will they stay? Although there exists research work shedding light on the job mobility problem at the organizational level, very few studies investigate the pattern at an individual level. To this end, in this work, I provide a hierarchical career-path-aware neural network method to understand the talents’ job mobility by learning their historical job-hopping behaviors. I am the first to solve two highly specific problems:
1) who will be the talents’ next employer? 2) how long will the talents stay at their new positions? In this framework, three different levels of information are considered, including personal-related information, company-related information, and position-related information. As we know, during one’s career path, one may experience several internal transfers within one company, as well as several external transfers among companies. A neural network model was designed to capture and understand those internal transfers and external transfers hierarchically, moreover, a delicate attention mechanism was implemented to obtain model interpretability. This model can effectively identify
both environmental and individual historical patterns that may influence the decision-making process of talents. More specifically, environmental factors refer to the connections among companies, while individual historical patterns reveal which experience from one’s historical career path plays the most important role in his/her future decision making. Different types of state-of-the-art methods are included for comparison, such as non-sequential model, sequential model, stochastic time series model and variants of our model. Our model is consistently superior to baselines with a large margin, demonstrating a strong predictive advantage. Moreover, several interesting patterns related to job mobility are discovered by examining the attention values. For instance, (1) the longer an individual stays with an employer, the higher attention (importance) the employer has; (2) a job appearing in a later position in one’s career path has higher attention; (3) in general, as the job duration increases, the importance of an employer increases as well, except for government-based organizations; (4) as the number of social connections increases, the attention increases as well; (5) people tend to stay with an employer for full years rather than leaving in the middle of a year. A summary of the preliminary work has been published in the
ACM SIGKDD Int’l Conf. on Knowledge Discovery and Data Mining (KDD 2019), which is the ranked first conference in the data mining field and highly selective with an acceptance rate of 14.2%.
Job Salary Benchmarking:
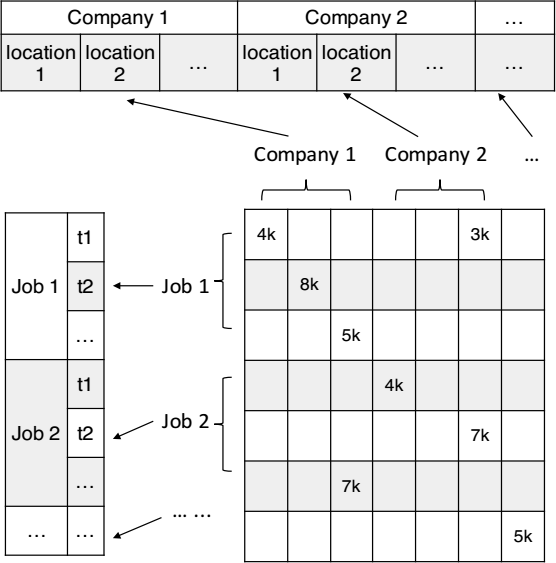
Job salary benchmarking
(JSB) is a process by which organizations acquire and analyze labor market data to determine appropriate compensation for their actual and prospective employees. As a fundamental tool for attracting, retaining, and motivating employees, salary benchmarking plays an important role in support of the success of a company's human resource management (e.g., maximizing the productivity of the company; minimizing the cost of human capital in a long-run view). “Good pay” is often viewed as the determinant reason for job seekers to accept their offers. The traditional salary benchmarking methods are largely based on limited survey data and statistical methods, and they suffer from un-inferable problems when the data are deficient. However, the lack of data will be the most common situation in the real world. Another problem is that most of the previous studies are job-category-based, which is too general to meet the particular requirement of Compensation and Benefit (C&B) department. Thus, a lot of manual expert tuning work is needed to adjust the final numbers, which is time-consuming and expensive. To this end, a fine-grained, automatic salary benchmarking system is needed by the organization. In this work, we first construct an expanded matrix where each row represents a time-specific job, while each column represents a location-specific company, and each entry is the corresponding salary. Thus the problem is formulated as a matrix completion problem, which a Matrix Factorization (MF) method can deal with. Next, the following four domain-related assumptions are first tested then integrated into the framework to improve the estimation efficiency: 1) If jobs have similar job responsibilities, their salaries should be closer. 2) If jobs are opened by two similar companies, their salaries should be closer. 3) If the jobs are wanted within a near period, their salaries should be closer. 4) If the work locations for the two jobs have similar economic conditions, their salaries should be closer. Based on the four observations, we designed four corresponding regularizers to optimize the learning process of the basic MF model. Our model was tested on a large-scale real-world online recruiting advertisement data, and compared with several state-of-art methods, the results show our model has consistently better performance. A summary of the preliminary work has been published in
IEEE Conference on Data Mining (ICDM 2018) as a full paper, where the acceptance rate is 11.1%.
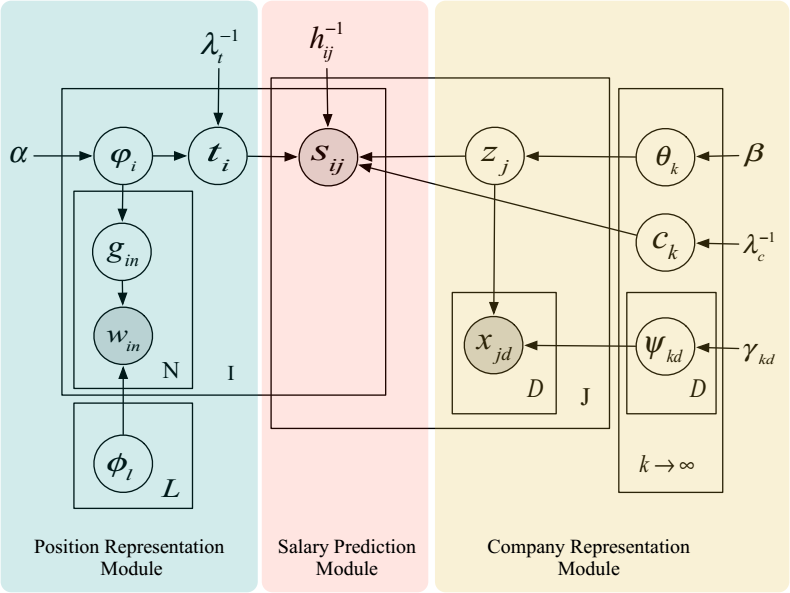
While the MF-based intelligent salary benchmarking model can effectively estimate the job’s salary with confounding factors, two main issues remain unsolved. It suffers from the “cold start” issue for a new company or job position, and limited model interpretability. Considering an extreme case: a new startup opens a data scientist position, and we cannot profile the company’s tendency with its historical records, while the employer still hopes to get a reliable scheme for the hiring process. In addition, the interpretable insights on prediction results are appreciated for helping an inexperienced recruiter understand the final decision. Along this line, I designed a Nonparametric Dirichlet-Process-based Latent Factor Model for JSB, namely
NDP-JSB, which can jointly model the latent representations of both company and job position, and predict job salaries for each company and job position with rich context. In this model, we first utilize a company representation module to classify location-specific companies into different clusters by assuming that companies in the same clusters share the same latent parameters. Meanwhile, we apply a position representation module to learn the corresponding job latent parameters based on their job descriptions. After that, we cross multiply the job latent parameters by the company latent parameters for the final salary predictions. In this way, we solved the issues we mentioned above. First, if a location-specific company is new to our database, we can still make the predictions. Second, our model can also provide interpretability of the relevant skillsets emphasized by each respective job. We compared this model with other baselines and the results verified the superiority of our framework. This work is in progress on the last phase and we plan to submit it as an article for the INFORMS Journal on Computing.